Beyond the AI Apocalypse: Rethinking How We Forecast Existential Risks
Why the most cited AI risk survey might be leading us astray, and what we can do about it.
Picture a world teetering on the precipice of transformation, where artificial intelligence holds the power to catapult humanity into a utopian future or usher in our extinction. Now, consider this: the prophets of this fate are not philosophers, ethicists, or social scientists, but computer scientists and machine learning researchers.
Welcome to the realm of AI risk predictions, where the Expert Survey on Progress in AI (ESPAI) reigns supreme. This survey, currently in its third iteration, has become the oracle of choice for those seeking to quantify our collective fear about AI's future. Media pundits parrot its findings, politicians wield its statistics in policy debates, and AI alarmists clutch it like a holy text.
But here's the crux: while these researchers are undoubtedly brilliant in their field, are they indeed the oracles we need to predict AI's impact on humanity's fate? The answer may not be as straightforward as we've been led to believe.
As someone who has spent decades in risk assessment, I can't help but see an elephant—or rather, a gorilla—in the room. I call it the "Invisible Gorilla" of AI risk forecasts: a pervasive assumption shaping our view of the future in ways we might not even realize. This assumption, as we'll explore, could be leading us astray in our attempts to navigate the complex landscape of AI risks.
Don't get me wrong. The ESPAI survey offers a valuable glimpse into the minds of those at the vanguard of AI development. However, when it comes to existential risks and societal upheavals, relying solely on these experts is akin to asking blockchain developers to forecast the future of global finance based on their mastery of cryptocurrency code.
In this article, I'll explain why we should approach these expert predictions with a healthy dose of skepticism, explore the hidden biases in how we interpret them, and suggest more effective ways to think about the future of AI. When it comes to the fate of humanity, maybe we need a little more forecasting and Risk Judgment and Decision-Making (RJDM) expertise.
The Silicon Valley Crystal Ball
Unless you've been living under a digital rock, you've likely encountered this eye-popping statistic: "Half of AI researchers believe there's a 10% or greater chance that humans will go extinct from our inability to control AI." This nugget of doomsday prophecy, birthed from the ESPAI survey, has proliferated through our collective consciousness with the virality of a cat video.
Consider Tristan Harris, the former Google design ethicist and co-founder of the Center for Humane Technology. He's been peddling a chilling analogy that's caught fire in the tech ethics sphere: "Imagine boarding a plane where half the engineers who built it warn you of a 10 percent chance it'll crash, killing everyone onboard. Would you still take that flight?"
It's a gut-punch of an image, isn’t it? It’s designed to short-circuit our rational thinking and tap directly into our fear centers. No wonder it's been echoed across YouTube videos, podcast interviews, and countless articles. Even Elon Musk has amplified this narrative, famously stating that AI is 'our biggest existential threat' and likening it to 'summoning the demon.
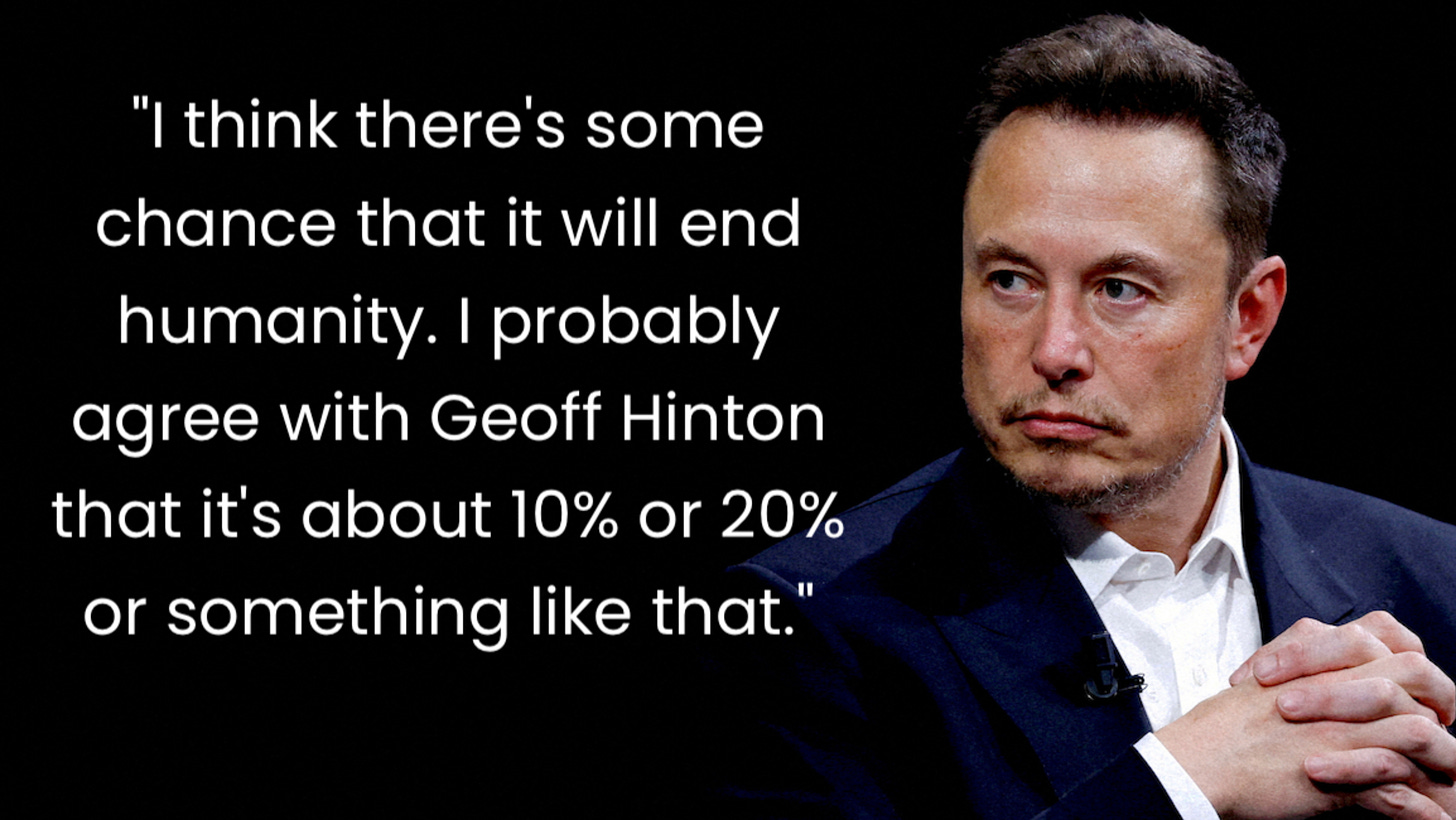
It's the kind of hyperbole that makes for great soundbites but questionable policy, and the implications of these claims extend far beyond social media debates and late-night talk shows. They're actively shaping real-world policies and business decisions, often without the critical examination such influential ideas warrant.
The influence of these survey results is evident in policy discussions from Capitol Hill to Downing Street, Brussels to Davos. Politicians and policymakers are invoking these findings to justify a wide range of actions, from proposing AI regulation bills to calling for international treaties on AI development. The survey has become a touchstone in debates about how to govern a technology that is still largely theoretical in its most advanced forms.
In a recent forum, US Senator Chuck Schumer asked participants to provide their "p(doom)" and "p(hope)") probabilities for artificial general intelligence (AGI).
Perhaps more concerningly, the US State Department commissioned and released a report warning of "extinction-level" threats to humanity from advanced AI systems. This report, based on interviews with a ‘selected sample’ of 200 AI experts and business leaders, is a tragic example of how these fallacies and the suspicious economic forces behind most of the AI doomerism can influence even those organizations whose very mission hangs on (risk) forecasting competency.
The ripple effects of these narratives extend beyond national borders. Both the United Nations and the European Union have accelerated their regulatory efforts, citing the need to address potential catastrophic outcomes. Dozens of other national and international initiatives have followed suit, many driven by similar existential risk narratives.
The ESPAI survey has thus transcended its origins as an academic exercise, igniting a classic availability cascade about AI risks. It has become a de facto oracle, consulted by tech moguls in Silicon Valley, financial analysts on Wall Street, and policymakers in Washington, all seeking to divine humanity's future in the age of AI.
But here's the million-dollar question: Is this collective crystal-gazing offering us genuine insight into our future, or is it merely evidence of our cognitive biases and risk judgment and forecasting ineptitude?
Unraveling the ESPAI Survey
Let me be clear: I'm not dismissing concerns about AI risks. On the contrary, there are numerous substantive near- and long-term risks that AI could generate or amplify. These range from job displacement and algorithmic bias to more speculative scenarios involving advanced AI systems.
What I am proposing, however, is that we need to critically examine how we articulate and measure these 'catastrophic' and so-called existential (or 'X') risks. And I'm not alone in calling for this kind of scrutiny.
Melanie Mitchell, a computer scientist and AI researcher, has been one of the most vocal critics. Her analysis highlights several fundamental issues that undermine the survey's reliability as a basis for policy decisions. For starters, the sample size for some of the most quoted questions was tiny. We're talking about basing world-changing decisions on the opinions of fewer people than you'd find in a small-town city council meeting.*
But it's not just about numbers. Mitchell also highlights the vague time horizon of the risk questions. Asking about the probability of human extinction without specifying a timeframe is like asking, "How likely is it that it will rain?" Without knowing if we're talking about tomorrow or next year, how can we interpret the answers?
Other experts have chimed in with their own concerns:
The "expert" problem: Who exactly are we calling experts here? The survey doesn't distinguish between a fresh-faced grad student and a seasoned researcher with decades of experience. Should we be weighing their opinions equally? (This objection, however close, is missing the key fallacy we address herein.)
The response bias: Who chose to answer these questions? Are we hearing from a representative sample, or just from those who have strong opinions on AI risk?
The confidence conundrum: How sure are these experts about their predictions? The survey doesn't tell us if someone is making an educated guess or speaking with high confidence.
These critiques aren't just academic nitpicking. They're fundamental issues that call into question the validity of using this survey as an AI risk barometer for humanity's future.
But here's the kicker: even with all these methodological red flags, the ESPAI survey continues to be cited as gospel truth. It's as if we're so desperate for answers about our AI-driven future that we're willing to overlook the flaws in how we're asking the questions.
And that brings us back to our Invisible Gorilla – the fundamental oversight that's been hiding in plain sight throughout this entire discourse.
The Invisible Gorilla: What We're All Missing
Picture this: You're watching a video of people passing basketballs. Your job is to count how many times the players in white shirts pass the ball. You focus intently, keeping careful count. At the end of the video, you're asked if you noticed anything unusual. "No," you confidently reply. Then you're shown the video again, and to your astonishment, a person in a gorilla suit walks right through the middle of the game, thumps their chest, and walks off. How did you miss that?
This famous psychological experiment, conducted by Daniel Simons and Christopher Chabris, demonstrates a phenomenon known as inattentional blindness. When we're focused on one task, we can become blind to even the most obvious things right in front of us.
I submit to you that we have an Invisible Gorilla in our AI risk assessment, and it's this: We're assuming that AI researchers are the best qualified to forecast the existential risks posed by AI.
Would we ask nuclear physicists to predict the geopolitical consequences of nuclear proliferation? Would we rely on geneticists to forecast the societal impacts of CRISPR technology? Probably not. We'd recognize that while their technical expertise is crucial, understanding the broader implications requires insights from political scientists, sociologists, economists, and a host of other disciplines.
Yet when it comes to AI, we're doing exactly that. We're asking the people who are heads-down in the code to lift their gaze and predict the fate of humanity. It's like asking a petroleum engineer specializing in hydraulic fracturing to estimate the technology’s impact on global climate or on the frequency and severity of catastrophic weather events in the next 100 years. They might have some interesting insights, but are they really the best equipped for that task?
The authors of the ESPAI survey, to their credit, acknowledge in their notes that forecasting is hard, even for experts. But then they proceed as if this caveat doesn't fundamentally undermine the entire exercise. It's a bit like saying, "This compass might not be accurate, but let's use it to set our course across the ocean anyway."
What's more, the further out we try to predict, the more tenuous our forecasts become. Predicting the direct, immediate impacts of AI? Sure, AI researchers might have valuable insights there. But when we start talking about second, third, or fourth-order consequences – the kind that could lead to existential risks – we're in uncharted territory. We're no longer talking about technology alone, but about its complex interactions with human psychology, social structures, economic systems, and more.
It's like asking a petroleum engineer specializing in hydraulic fracturing to estimate the technology’s impact on global climate…
By fixating on the technical expertise of AI researchers and being distracted by the enormity of the claims, we've become blind to the broader perspective and relevant expertise needed to truly grapple with these questions. We're counting basketballs while missing the gorilla dancing right in front of us. Who holds risk judgment competency? And how should (risk) forecasts be conducted?
Here's a litmus test: The next time an AI expert or business leader offers their "P-doom" estimates, ask them to predict their own mortality risk for the coming year. It's an astronomically simpler forecast, infinitely more personal, and instantly verifiable. Their response might tell you more about their risk judgment competency than any long-term AI forecast ever could.
How to Elevate the Discourse
So, what's the solution? It's not to disregard AI researchers' insights. Rather, it's to recognize their predictions' limitations and adopt RJDM and forecasting best practices.
To be clear, despite the efforts (and beliefs) of risk professionals and decision scientists, there is no universal or meta RJDM framework. There are dozens of useful risk management and decision-support methods, tools, and schools of thought, but - despite their claims - their practical value is in most cases inversely related to the breadth and scope of the decision space they emerge from.
This is why I have been advocating and teaching interdisciplinary RJDM competencies to senior decision-makers and risk, intelligence, and security professionals worldwide.
The personal RJDM space alone (what we call ‘safe-esteem’) demands a multitude of strategies and approaches depending on the domain's ‘validity’ (high vs. low), the velocity of the threat or hazard, available resources, personal values, etc.
Several proven strategies do exist for long-term, high-uncertainty forecasting efforts. Expert polls and surveys can be effective tools, but not in the form adopted by the ESPAI initiative.
Here’s a basic recipe:
Disambiguate
The survey and most ensuing conversations commit the cardinal sin of risk judgment: ambiguity. In order to have realistic and useful discussions about any risk, we need to identify what measurable phenomena we are estimating.
Consider this: A travel destination risk rating is utter nonsense (despite it being very popular) unless it measures (e.g. forecasts) the incidence of specific and unambiguous phenomena such as murder, road accidents, or communicable diseases.
It’s no surprise that the only AI risk domains where you can find intelligent and informed debates are in biochemical engineering, cybersecurity, and military (weaponization and battlefield) domains - where subject-matter experts are grounding (possible) futures to existing or future scenarios, vulnerabilities, and mitigation strategies. (There are plenty of other similar risk forecasts and constructive initiatives - from labor markets to learning and education - but I am focusing here on the catastrophic or existential risk.)
Embrace Scenario Planning
Rather than fixating on probabilities, let's explore a range of possible futures. This approach, used by everyone from military strategists to climate scientists, can help us prepare for multiple outcomes, not just the one we think is most likely. More importantly, scenarios don’t need to be exhaustive for specialists or domain experts to evaluate the credibility or likelihood of specific conditions, outcomes, or early warnings that emerge from these exercises.
A key element of effective scenario planning is establishing and monitoring milestones, key indicators, and triggers—all of which drive continuous adjustment and refinement of the process. This also encourages focusing on near-term developments and challenges, which is a much more effective way to ground the conversation, measure risks, and generate robust mitigation strategies.
Expand the Expert Pool and Enforce Training
Instead of just asking AI researchers, let's bring historians, anthropologists, economists, psychologists, and ethicists into the conversation.
During the early stages of the COVID-19 pandemic, the German government sought guidance from various scientific organizations. The initial response was focused on medical and epidemiological knowledge, but the authorities soon realized the necessity of a more comprehensive, interdisciplinary approach to tackle the complex challenges brought about by the crisis. In April 2020, Germany engaged scholars from the humanities to help bring about an end to the coronavirus lockdown and to facilitate the easing of restrictions. This diverse team comprised historians, philosophers, theologians, and jurists, among others.
Experts should receive training on the elicitation process, including an introduction to probability and uncertainty, heuristics and biases, and the specific subject matter at hand.
As we navigate the uncharted waters of AI development, we must resist the allure of simplistic doom prophecies or utopian promises. The path forward lies not in dismissing AI researchers' insights but in contextualizing them within a broader, more nuanced framework of risk assessment. By embracing interdisciplinary approaches, scenario planning, and rigorous training in risk evaluation, we can elevate the discourse surrounding AI's future impact.
Our goal should be to move beyond attention-grabbing headlines and foster a more sophisticated, reality-based understanding of both the potential and pitfalls of AI. Only then can we hope to shape policies and strategies that genuinely serve humanity's best interests in the face of this transformative technology. The stakes are too high for anything less than our most clear-eyed, comprehensive approach to navigating our AI-infused future.
* The 2023 ESPAI attempts to address some of the criticism levied against it by Mitchell and others, such as the conflation issue, by asking more specific questions about extinction risks. However, it does not directly resolve the problem of language ambiguity, as it still relies on terms open to interpretation without providing explicit definitions.